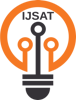
International Journal on Science and Technology
E-ISSN: 2229-7677
•
Impact Factor: 9.88
A Widely Indexed Open Access Peer Reviewed Multidisciplinary Bi-monthly Scholarly International Journal
Plagiarism is checked by the leading plagiarism checker
Call for Paper
Volume 16 Issue 2
2025
Indexing Partners

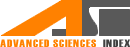
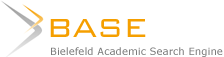

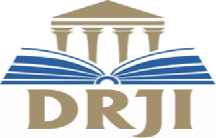

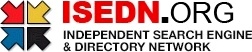

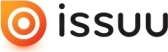

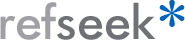
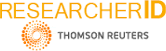
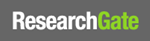
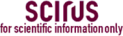
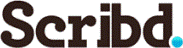

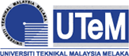
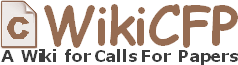
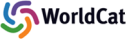
Predicting Individual Radiosenstivity Based on Telomere Length Using Hybrid Deep Learning Models
Author(s) | MD Shakeel Ahmed, Shaik Safeena, Shaik Reshma, SP Uma Maheswari |
---|---|
Country | India |
Abstract | While radiotherapy is critically important for treating cancer, it is observed that patients tend to respond differently based on their genetics such as telomere length, which happens to be a crucial element of radiosensitivity. While it is known that shorter telomeres have greater sensitivity to radiation, predicting a person's response using only this metric is not possible. The objective of this project is to create a predictive model for radiosensitivity through hybrid deep learning approaches. Classical models, e.g., decision trees or linear regression, are not able to model intricate relationships in genomic data, especially when combining multiple features. The suggested method features the integration of Convolutional Neural Networks (CNNs), Long Short-Term Memory (LSTM) networks, and Autoencoders. CNNs extract spatial features in telomere sequence data, LSTMs learn temporal patterns, and Autoencoders compress data dimensionality and learn latent features. By combining these models, the method enhances prediction precision and offers a more complete assessment of radiosensitivity. This might result in more tailored radiotherapy, maximizing treatment planning and improving clinical decision-making in cancer therapy |
Keywords | Radiosensitivity, Telomere Length, Hybrid Deep Learning, CNN, LSTM, LGBM Classifier, Tkinter, Classification. |
Field | Engineering |
Published In | Volume 16, Issue 2, April-June 2025 |
Published On | 2025-04-11 |
Cite This | Predicting Individual Radiosenstivity Based on Telomere Length Using Hybrid Deep Learning Models - MD Shakeel Ahmed, Shaik Safeena, Shaik Reshma, SP Uma Maheswari - IJSAT Volume 16, Issue 2, April-June 2025. DOI 10.71097/IJSAT.v16.i2.2512 |
DOI | https://doi.org/10.71097/IJSAT.v16.i2.2512 |
Short DOI | https://doi.org/g9fchj |
Share this

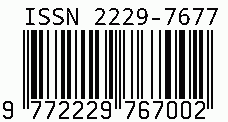
CrossRef DOI is assigned to each research paper published in our journal.
IJSAT DOI prefix is
10.71097/IJSAT
Downloads
All research papers published on this website are licensed under Creative Commons Attribution-ShareAlike 4.0 International License, and all rights belong to their respective authors/researchers.
