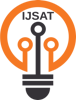
International Journal on Science and Technology
E-ISSN: 2229-7677
•
Impact Factor: 9.88
A Widely Indexed Open Access Peer Reviewed Multidisciplinary Bi-monthly Scholarly International Journal
Plagiarism is checked by the leading plagiarism checker
Call for Paper
Volume 16 Issue 2
2025
Indexing Partners

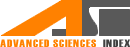
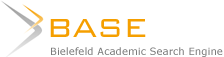

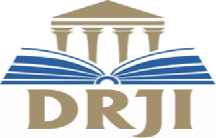

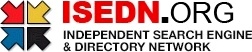

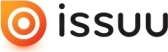

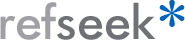
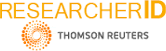
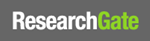
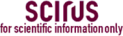
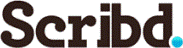

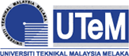
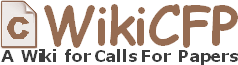
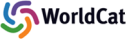
Machine Learning for Fraud Detection in Financial Transactions
Author(s) | Srinivasa Kalyan Vangibhurathachhi |
---|---|
Country | United States |
Abstract | As the global financial systems become more globalized and digitized, the threats of financial fraud are becoming massive accounting over $5 trillion annually, and sophisticated as cyber criminals ever-changing tactics. The traditional rule-based fraud detection systems that institutions have relied on are unable to meet this escalating challenge due to their inflexibility, high false-positive rates, and inability to process large-scale data in real-time. Machine learning offers an impressive alternative with transformative solutions that leverage predictive analytics models, anomaly detection, and adaptive learning to boost systems’ accuracy, scalability, and responsiveness in fraud detection. This article investigates the application of supervised, unsupervised, and deep learning machine learning techniques in detecting credit card fraud, identity theft, and cryptocurrency scams, highlighting their advantages over conventional methods. The article also highlights the challenges that machine learning techniques need to grapple with through more research and investment to be more efficient, such challenges include data imbalance, regulatory constraints, computational costs, and model interpretability. |
Field | Engineering |
Published In | Volume 16, Issue 1, January-March 2025 |
Published On | 2025-03-05 |
Cite This | Machine Learning for Fraud Detection in Financial Transactions - Srinivasa Kalyan Vangibhurathachhi - IJSAT Volume 16, Issue 1, January-March 2025. DOI 10.71097/IJSAT.v16.i1.3727 |
DOI | https://doi.org/10.71097/IJSAT.v16.i1.3727 |
Short DOI | https://doi.org/g9fmwc |
Share this

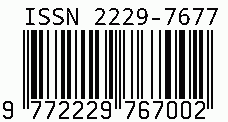
CrossRef DOI is assigned to each research paper published in our journal.
IJSAT DOI prefix is
10.71097/IJSAT
Downloads
All research papers published on this website are licensed under Creative Commons Attribution-ShareAlike 4.0 International License, and all rights belong to their respective authors/researchers.
