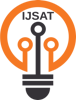
International Journal on Science and Technology
E-ISSN: 2229-7677
•
Impact Factor: 9.88
A Widely Indexed Open Access Peer Reviewed Multidisciplinary Bi-monthly Scholarly International Journal
Plagiarism is checked by the leading plagiarism checker
Call for Paper
Volume 16 Issue 2
2025
Indexing Partners

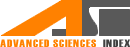
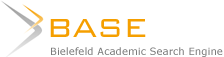

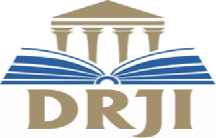

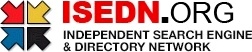

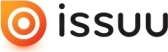

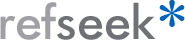
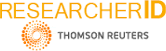
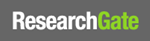
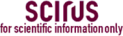
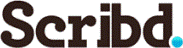

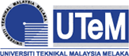
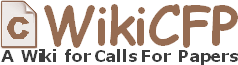
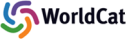
Deep CNN Based Multi-Class Retinal Disease Detection with Reduced Memory Overhead
Author(s) | A.Jahnavi, S.Yashwanth Kumar, E.Sophia Raj, M.Tejaswi, S.Krishnakumar |
---|---|
Country | India |
Abstract | Extensive research directions for important disease diagnosis and classification includes ANN, Deep learning, RNN, Alex Net, and ResNet. Retinal disease classification has been transformed by CNN and U-Net Segmentation. The intricacy of feature extraction causes U-Net to pass the entire feature map to the decoder, which causes errors related to memory and CPU. It stops pooling index reuse when combined with the unsampled decoder feature map. For problems involving multiple classes of data, this study presents a convolutional neural network (CNN) model that makes optimal use of memory. The suggested model was evaluated using an Eye Net benchmark dataset that contained 32 different forms of retinal illnesses. The proposed model enhances memory management and precision, according to the experimental results. In order to compare accuracy, precision, and recall, various epochs and step timings were utilized. The suggested approach performed well on Eye-net. |
Keywords | Classification, CNN, deep learning, Eye-Net, retina, U-Net |
Field | Computer > Artificial Intelligence / Simulation / Virtual Reality |
Published In | Volume 16, Issue 1, January-March 2025 |
Published On | 2025-03-31 |
Cite This | Deep CNN Based Multi-Class Retinal Disease Detection with Reduced Memory Overhead - A.Jahnavi, S.Yashwanth Kumar, E.Sophia Raj, M.Tejaswi, S.Krishnakumar - IJSAT Volume 16, Issue 1, January-March 2025. DOI 10.71097/IJSAT.v16.i1.3086 |
DOI | https://doi.org/10.71097/IJSAT.v16.i1.3086 |
Short DOI | https://doi.org/g9dgpv |
Share this

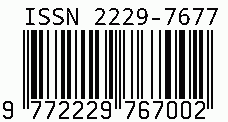
CrossRef DOI is assigned to each research paper published in our journal.
IJSAT DOI prefix is
10.71097/IJSAT
Downloads
All research papers published on this website are licensed under Creative Commons Attribution-ShareAlike 4.0 International License, and all rights belong to their respective authors/researchers.
