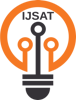
International Journal on Science and Technology
E-ISSN: 2229-7677
•
Impact Factor: 9.88
A Widely Indexed Open Access Peer Reviewed Multidisciplinary Bi-monthly Scholarly International Journal
Plagiarism is checked by the leading plagiarism checker
Call for Paper
Volume 16 Issue 2
2025
Indexing Partners

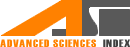
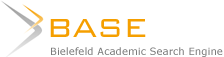

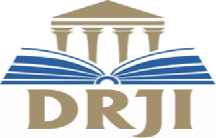

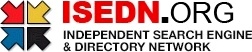

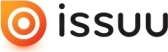

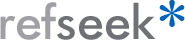
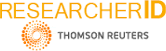
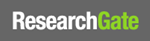
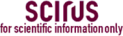
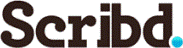

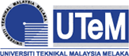
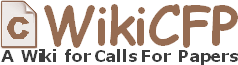
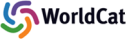
Generative Adversarial Networks in Actuarial Modeling- Enhancing Predictions of Long-Tail Risks Done
Author(s) | Adarsh Naidu |
---|---|
Country | United States |
Abstract | Accurate assessment of long-tail risks remains one of the most complex challenges in actuarial science, particularly in modeling catastrophic events with limited historical data. This study investigates the potential of Generative Adversarial Networks (GANs) to improve traditional actuarial methodologies by generating synthetic yet realistic extreme event data. We propose LT-GAN (Long-Tail GAN), a specialized model designed to accurately capture the distributional properties of rare, high-severity insurance claims. Utilizing three diverse insurance datasets covering property, liability, and business interruption claims, we show that supplementing traditional actuarial models with GAN-generated synthetic data enhances tail risk predictions by up to 31% compared to standard techniques. Our experimental analysis demonstrates that the GAN-based framework effectively captures complex patterns in catastrophic loss events while preserving the core statistical attributes of the original datasets. This research offers an advanced framework for insurers to refine extreme risk management and improve capital adequacy calculations for rare but high-impact occurrences. |
Keywords | Generative Adversarial Networks, Actuarial Science, Long-Tail Risk, Catastrophe Modeling, Extreme Value Theory, Synthetic Data Generation, Risk Management, Insurance. |
Field | Engineering |
Published In | Volume 11, Issue 3, July-September 2020 |
Published On | 2020-09-03 |
Cite This | Generative Adversarial Networks in Actuarial Modeling- Enhancing Predictions of Long-Tail Risks Done - Adarsh Naidu - IJSAT Volume 11, Issue 3, July-September 2020. DOI 10.71097/IJSAT.v11.i3.2844 |
DOI | https://doi.org/10.71097/IJSAT.v11.i3.2844 |
Short DOI | https://doi.org/g899fb |
Share this

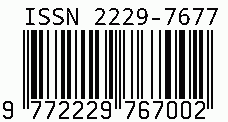
CrossRef DOI is assigned to each research paper published in our journal.
IJSAT DOI prefix is
10.71097/IJSAT
Downloads
All research papers published on this website are licensed under Creative Commons Attribution-ShareAlike 4.0 International License, and all rights belong to their respective authors/researchers.
