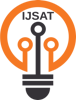
International Journal on Science and Technology
E-ISSN: 2229-7677
•
Impact Factor: 9.88
A Widely Indexed Open Access Peer Reviewed Multidisciplinary Bi-monthly Scholarly International Journal
Plagiarism is checked by the leading plagiarism checker
Call for Paper
Volume 16 Issue 2
2025
Indexing Partners

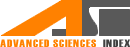
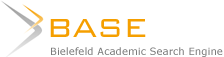

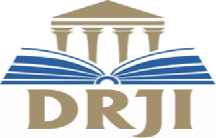

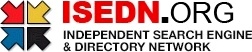

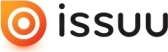

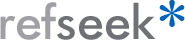
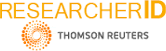
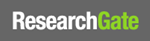
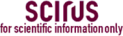
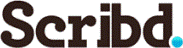

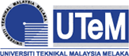
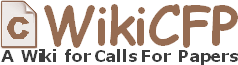
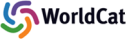
How AI Learns Hidden Patterns: The Power of Embeddings in Predictions
Author(s) | Huzaifa Fahad Syed |
---|---|
Country | United States |
Abstract | Embedding techniques have revolutionized artificial intelligence by enabling machines to understand complex relationships in data through dense numerical representations. This article explores the technical foundations of embeddings, tracing their evolution from word-level models like Word2Vec and GloVe to more sophisticated approaches like FastText and Sentence-BERT. The article examines how embeddings learn directly from data, capturing semantic and syntactic relationships in high-dimensional vector spaces. It demonstrates their practical applications across recommendation systems, healthcare, financial fraud detection, and natural language processing, where they consistently outperform traditional methods. The article also addresses computational challenges associated with large-scale embedding models and highlights advanced methods that balance performance with efficiency. The article emphasizes how embeddings have fundamentally transformed prediction systems by enabling machines to discover hidden patterns in data without explicit programming, establishing them as an essential component of modern AI systems. |
Keywords | Vector representations, semantic similarity, neural language models, recommendation systems, fraud detection |
Field | Computer |
Published In | Volume 16, Issue 1, January-March 2025 |
Published On | 2025-03-21 |
Cite This | How AI Learns Hidden Patterns: The Power of Embeddings in Predictions - Huzaifa Fahad Syed - IJSAT Volume 16, Issue 1, January-March 2025. DOI 10.71097/IJSAT.v16.i1.2596 |
DOI | https://doi.org/10.71097/IJSAT.v16.i1.2596 |
Short DOI | https://doi.org/g892gf |
Share this

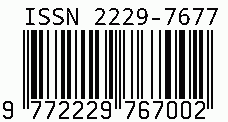
CrossRef DOI is assigned to each research paper published in our journal.
IJSAT DOI prefix is
10.71097/IJSAT
Downloads
All research papers published on this website are licensed under Creative Commons Attribution-ShareAlike 4.0 International License, and all rights belong to their respective authors/researchers.
