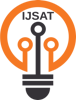
International Journal on Science and Technology
E-ISSN: 2229-7677
•
Impact Factor: 9.88
A Widely Indexed Open Access Peer Reviewed Multidisciplinary Bi-monthly Scholarly International Journal
Plagiarism is checked by the leading plagiarism checker
Call for Paper
Volume 16 Issue 2
2025
Indexing Partners

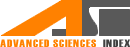
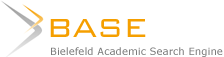

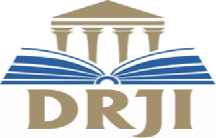

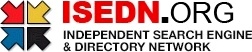

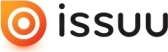

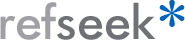
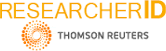
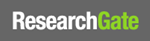
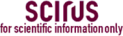
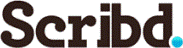

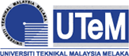
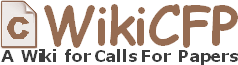
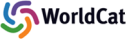
Augmenting Small Datasets With Synthetic Data For Data Science Models
Author(s) | Prathmesh Raut, Atharva Samindre, Ashish Velhal |
---|---|
Country | India |
Abstract | In machine learning, high-quality datasets are essential for accurate predictions, but many fields like healthcare and finance face data scarcity, imbalance, and collection challenges, leading to poor model performance. Synthetic data mimics real-world data and has emerged as a solution to augment small datasets, improve diversity, and address underrepresented classes. It also mitigates privacy concerns in sensitive domains. Techniques like Generative Adversarial Networks (GANs) use a generator and discriminator to create realistic synthetic data, while Variational Autoencoders (VAEs) encode and decode data to generate new points. Methods like SMOTE address class imbalances by creating synthetic samples. These advancements enable better model performance without relying solely on costly or hard-to-collect real-world data, benefiting critical applications in healthcare, finance, and beyond. |
Keywords | Synthetic Data, Data Augmentation, GANs, VAEs, Machine Learning, Data Scarcity |
Field | Computer > Data / Information |
Published In | Volume 16, Issue 1, January-March 2025 |
Published On | 2025-03-21 |
Cite This | Augmenting Small Datasets With Synthetic Data For Data Science Models - Prathmesh Raut, Atharva Samindre, Ashish Velhal - IJSAT Volume 16, Issue 1, January-March 2025. DOI 10.71097/IJSAT.v16.i1.2565 |
DOI | https://doi.org/10.71097/IJSAT.v16.i1.2565 |
Short DOI | https://doi.org/g892gr |
Share this

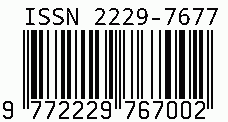
CrossRef DOI is assigned to each research paper published in our journal.
IJSAT DOI prefix is
10.71097/IJSAT
Downloads
All research papers published on this website are licensed under Creative Commons Attribution-ShareAlike 4.0 International License, and all rights belong to their respective authors/researchers.
