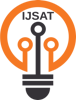
International Journal on Science and Technology
E-ISSN: 2229-7677
•
Impact Factor: 9.88
A Widely Indexed Open Access Peer Reviewed Multidisciplinary Bi-monthly Scholarly International Journal
Plagiarism is checked by the leading plagiarism checker
Call for Paper
Volume 16 Issue 2
2025
Indexing Partners

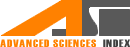
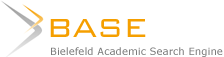

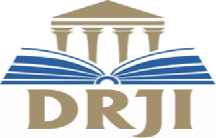

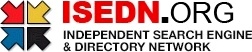

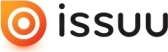

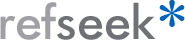
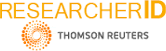
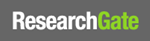
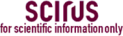
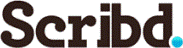

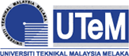
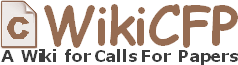
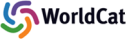
Neuroimage Analysis For Stroke Detection
Author(s) | Matta Smyla, Vadugu Rajeswari, Pedakotla Kusuma Kiran Venkata Sai, Kamatam Moses Bhagya Raj, Chandana Suresh |
---|---|
Country | India |
Abstract | An abstract is a concise version of the full paper. A rapid and accurate stroke detection mechanism thus remains the key to treating strokes and improving patient outcomes. Traditional methods of stroke diagnosis are often plagued with their own sets of problems regarding accuracy and speed. The present study describes a new diagnosis approach in which machine learning techniques are used to classify neuroimages into normal and stroke-pathology categories in the framework of DenseNet. The DenseNet architecture, characterized by dense connections, enhances this diagnostic approach by allowing for a better gradient flow and refinement in feature extraction. Our system exposes the DenseNet framework to heterogeneous neuro-images-advanced preprocessing approaches that are utilized to improve the overall adaptability and performance. Early results indicate that the DenseNet model is capable of achieving a respectable test accuracy of 96.60% and training accuracy of 99% when normal neuro-images are used for training. To compare its performance, accuracies of other architectures were also evaluated: the CNN model attained accuracy with 86%, MobileNet scored 92%, and ResNet achieved 93.60%. Such comparisons place DenseNet way ahead in terms of potentially increasing the accuracy and reliability of stroke detection. Thus, this diagnostic tool can be a great help for health practitioners. The next step in the road map includes validating the model on larger datasets and conducting clinical studies to check how effective it is when deployed in practical scenarios. This study shows how deep learning models can revolutionize stroke diagnosis and, ultimately, patient management. |
Field | Engineering |
Published In | Volume 16, Issue 1, January-March 2025 |
Published On | 2025-03-16 |
Cite This | Neuroimage Analysis For Stroke Detection - Matta Smyla, Vadugu Rajeswari, Pedakotla Kusuma Kiran Venkata Sai, Kamatam Moses Bhagya Raj, Chandana Suresh - IJSAT Volume 16, Issue 1, January-March 2025. DOI 10.71097/IJSAT.v16.i1.2454 |
DOI | https://doi.org/10.71097/IJSAT.v16.i1.2454 |
Short DOI | https://doi.org/g88sbj |
Share this

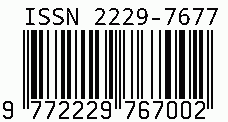
CrossRef DOI is assigned to each research paper published in our journal.
IJSAT DOI prefix is
10.71097/IJSAT
Downloads
All research papers published on this website are licensed under Creative Commons Attribution-ShareAlike 4.0 International License, and all rights belong to their respective authors/researchers.
