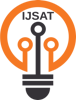
International Journal on Science and Technology
E-ISSN: 2229-7677
•
Impact Factor: 9.88
A Widely Indexed Open Access Peer Reviewed Multidisciplinary Bi-monthly Scholarly International Journal
Plagiarism is checked by the leading plagiarism checker
Call for Paper
Volume 16 Issue 2
2025
Indexing Partners

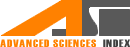
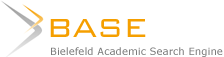

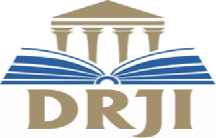

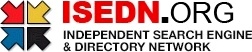

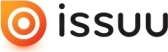

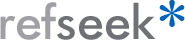
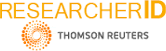
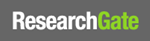
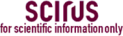
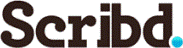

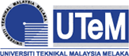
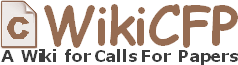
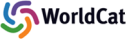
Artificial Intelligence-Driven Business Intelligence: Machine Learning Techniques for Financial Market Analysis
Author(s) | Md Atiqur Rahman, Md Hasan khan, Saidul Islam, Kazi Siam Al Mobin, Md Firoz Kabir |
---|---|
Country | India |
Abstract | The accelerated development of Artificial Intelligence (AI) and Machine Learning (ML) has revolutionised financial market analysis by improving predictive accuracy, risk assessment, and algorithmic trading strategies. This paper introduces a business intelligence framework that is AI-driven and incorporates a variety of machine learning techniques to enhance sentiment analysis, risk management, and stock price forecasting in financial markets. We utilise Long Short-Term Memory (LSTM) networks, Autoregressive Integrated Moving Average (ARIMA), and XGBoost to forecast stock prices, attaining Root Mean Squared Error (RMSE) values as low as 1.52. This represents an improvement in accuracy over conventional models. Monte Carlo Simulations, Bayesian Networks, and Value at Risk (VaR) methods are employed for financial risk assessment. These methods demonstrate a reduction in Maximum Drawdown (MDD) from -15% (traditional models) to -8% (AI models) and an improvement in Sharpe Ratio from 0.8 to 1.2, which suggests improved risk-adjusted profits. In addition, we employ Natural Language Processing (NLP) models, such as BERT and LSTMs, to analyse financial sentiment from 50,000 social media posts and 10,000 news articles. Our sentiment classification approach achieves an F1-score of 80%, which is a significant improvement over conventional sentiment models (F1-score 62%). Additionally, algorithmic trading strategies that are based on reinforcement learning exhibit a trading success rate of 85%, with a 20.3% increase in profitability compared to traditional trading strategies (12.1%). These findings emphasise the potential of AI to improve financial intelligence by enhancing predictive accuracy, risk management, and decision-making. Although progress has been made, there are still significant obstacles to overcome, including regulatory compliance, data integrity, and model interpretability. The research also investigates potential future directions, such as the integration of AI and blockchain technology, the use of quantum computing for financial simulations, and the implementation of AI-driven ESG (Environmental, Social, and Governance) investing. This study offers a comprehensive AI-driven financial analysis model that features highly effective, data-driven decision-making tools for policymakers, financial analysts, and investors. |
Keywords | Artificial Intelligence in Finance,Machine Learning for Financial Markets,Stock Price Prediction,Risk Assessment and Management,Algorithmic Trading Strategies |
Field | Computer > Artificial Intelligence / Simulation / Virtual Reality |
Published In | Volume 16, Issue 1, January-March 2025 |
Published On | 2025-03-13 |
Cite This | Artificial Intelligence-Driven Business Intelligence: Machine Learning Techniques for Financial Market Analysis - Md Atiqur Rahman, Md Hasan khan, Saidul Islam, Kazi Siam Al Mobin, Md Firoz Kabir - IJSAT Volume 16, Issue 1, January-March 2025. DOI 10.71097/IJSAT.v16.i1.2420 |
DOI | https://doi.org/10.71097/IJSAT.v16.i1.2420 |
Short DOI | https://doi.org/g88sb2 |
Share this

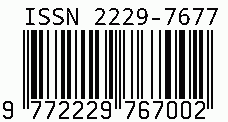
CrossRef DOI is assigned to each research paper published in our journal.
IJSAT DOI prefix is
10.71097/IJSAT
Downloads
All research papers published on this website are licensed under Creative Commons Attribution-ShareAlike 4.0 International License, and all rights belong to their respective authors/researchers.
