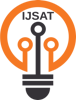
International Journal on Science and Technology
E-ISSN: 2229-7677
•
Impact Factor: 9.88
A Widely Indexed Open Access Peer Reviewed Multidisciplinary Bi-monthly Scholarly International Journal
Plagiarism is checked by the leading plagiarism checker
Call for Paper
Volume 16 Issue 2
2025
Indexing Partners

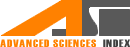
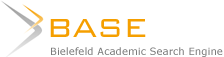

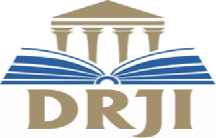

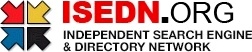

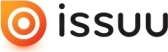

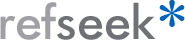
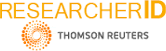
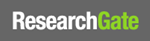
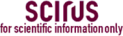
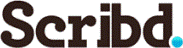

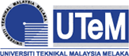
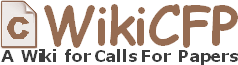
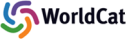
Sleep Disorder Prediction using Advanced Machine Learning Techniques
Author(s) | Mrs. P. Sarala, Ch. Charitha, Ch. Kusuma Kala, A. Yaswanthi, Abdul Haq |
---|---|
Country | India |
Abstract | Sleep disorders like insomnia, sleep apnea, and restless legs syndrome significantly impact global health, increasing risks of cardiovascular diseases, cognitive decline, and reduced quality of life. Traditional diagnostic methods, such as polysomnography (PSG), are costly, resource-intensive, and require clinical monitoring. This study proposes a machine learning-based predictive model as a non-invasive, cost-effective, and accurate alternative for sleep disorder detection. By integrating supervised learning, ensemble methods, and recurrent neural networks (RNNs), the model analyzes physiological signals, medical history, sleep patterns, and demographic data. Comparative analysis shows that deep learning models, particularly those handling temporal data, achieve superior predictive accuracy. Future work will focus on incorporating diverse data sources, validating larger datasets, and exploring real-time applications for home-based and clinical monitoring. |
Keywords | prediction of sleep disorders, detection of sleep apnea, classification of insomnia, neural networks (RNN, LSTM),ensemble learning, and evaluation of sleep health. |
Field | Computer > Data / Information |
Published In | Volume 16, Issue 1, January-March 2025 |
Published On | 2025-03-11 |
Cite This | Sleep Disorder Prediction using Advanced Machine Learning Techniques - Mrs. P. Sarala, Ch. Charitha, Ch. Kusuma Kala, A. Yaswanthi, Abdul Haq - IJSAT Volume 16, Issue 1, January-March 2025. DOI 10.71097/IJSAT.v16.i1.2182 |
DOI | https://doi.org/10.71097/IJSAT.v16.i1.2182 |
Short DOI | https://doi.org/g87rhp |
Share this

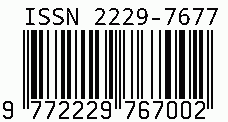
CrossRef DOI is assigned to each research paper published in our journal.
IJSAT DOI prefix is
10.71097/IJSAT
Downloads
All research papers published on this website are licensed under Creative Commons Attribution-ShareAlike 4.0 International License, and all rights belong to their respective authors/researchers.
