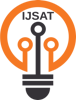
International Journal on Science and Technology
E-ISSN: 2229-7677
•
Impact Factor: 9.88
A Widely Indexed Open Access Peer Reviewed Multidisciplinary Bi-monthly Scholarly International Journal
Plagiarism is checked by the leading plagiarism checker
Call for Paper
Volume 16 Issue 2
2025
Indexing Partners

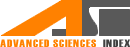
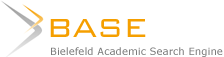

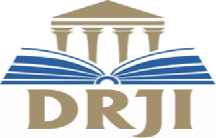

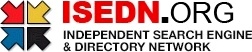

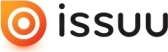

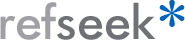
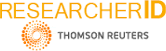
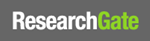
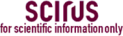
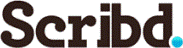

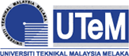
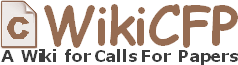
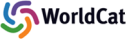
Integrating Sentiment Analysis and Topic Modeling for Social License to Operate
Author(s) | Syed Arham Akheel, Tarun Shrivas |
---|---|
Country | United States |
Abstract | Social License to Operate (SLO) refers to the informal acceptance or approval that organizations receive from their stakeholders and the broader community. In recent years, sentiment analysis and topic modeling have emerged as powerful tools for understanding the nuances of community perceptions in real time. This literature review examines the technical foundations of sentiment analysis and Latent Dirichlet Allocation (LDA)-based topic modeling, and explores how their hybrid integration can provide richer insights into stakeholder sentiments relevant to SLO. By analyzing scholarly works that investigate machine learning algorithms, natural language processing (NLP) techniques, and case studies across various industries, this paper synthesizes the current methodological landscape and identifies emerging trends and knowledge gaps. The findings underscore the importance of robust data governance, interdisciplinary collaboration, and ethical considerations for effectively deploying hybrid models to maintain and strengthen an organization’s Social License to Operate. |
Keywords | Social License to Operate, Sentiment Analysis, Topic Modeling, Latent Dirichlet Allocation, Hybrid Approaches, Natural Language Processing |
Field | Engineering |
Published In | Volume 12, Issue 2, April-June 2021 |
Published On | 2021-06-08 |
Cite This | Integrating Sentiment Analysis and Topic Modeling for Social License to Operate - Syed Arham Akheel, Tarun Shrivas - IJSAT Volume 12, Issue 2, April-June 2021. DOI 10.5281/zenodo.14866293 |
DOI | https://doi.org/10.5281/zenodo.14866293 |
Short DOI | https://doi.org/g84xm8 |
Share this

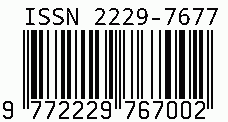
CrossRef DOI is assigned to each research paper published in our journal.
IJSAT DOI prefix is
10.71097/IJSAT
Downloads
All research papers published on this website are licensed under Creative Commons Attribution-ShareAlike 4.0 International License, and all rights belong to their respective authors/researchers.
