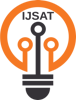
International Journal on Science and Technology
E-ISSN: 2229-7677
•
Impact Factor: 9.88
A Widely Indexed Open Access Peer Reviewed Multidisciplinary Bi-monthly Scholarly International Journal
Plagiarism is checked by the leading plagiarism checker
Call for Paper
Volume 16 Issue 3
July-September 2025
Indexing Partners

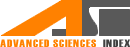
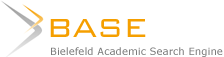

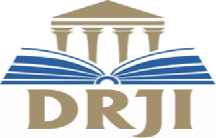

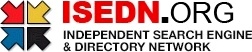

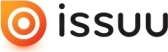

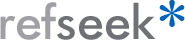
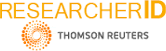
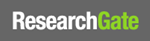
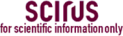
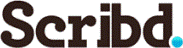

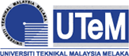
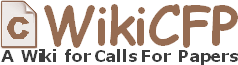
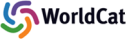
A Comprehensive Review of Cross-Validation Techniques in Machine Learning
Author(s) | Meenu Bhagat, Dr. Brijesh Bakariya |
---|---|
Country | India |
Abstract | In order to make sure that machine learning models are reliable and broadly applicable, cross-validation approaches are essential. They offer a methodical approach for adjusting hyperparameters, assessing model performance, and resolving issues with overfitting, unbalanced data, and temporal dependencies. This review article provides a thorough analysis of the many cross-validation strategies used in machine learning, from conventional techniques like k-fold cross-validation to more specialized strategies for particular kinds of data and learning objectives. In addition to current developments and best practices in cross-validation methodology, we go over the fundamentals, uses, benefits, and drawbacks of each technique. We also highlight important factors to take into account and recommendations for choosing suitable cross-validation procedures based on the properties of the dataset and the modelling goals. The objective of this study is to give academics and practitioners a thorough grasp of cross-validation approaches and their significance in developing robust and dependable machine learning models by synthesizing the available literature. |
Field | Computer |
Published In | Volume 16, Issue 1, January-March 2025 |
Published On | 2025-01-03 |
DOI | https://doi.org/10.71097/IJSAT.v16.i1.1305 |
Short DOI | https://doi.org/g82pcx |
Share this

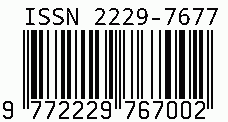
CrossRef DOI is assigned to each research paper published in our journal.
IJSAT DOI prefix is
10.71097/IJSAT
Downloads
All research papers published on this website are licensed under Creative Commons Attribution-ShareAlike 4.0 International License, and all rights belong to their respective authors/researchers.
