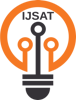
International Journal on Science and Technology
E-ISSN: 2229-7677
•
Impact Factor: 9.88
A Widely Indexed Open Access Peer Reviewed Multidisciplinary Bi-monthly Scholarly International Journal
Home
Research Paper
Submit Research Paper
Publication Guidelines
Publication Charges
Upload Documents
Track Status / Pay Fees / Download Publication Certi.
Editors & Reviewers
View All
Join as a Reviewer
Reviewer Referral Program
Get Membership Certificate
Current Issue
Publication Archive
Conference
Contact Us
Plagiarism is checked by the leading plagiarism checker
Call for Paper
Volume 16 Issue 1
2025
Indexing Partners

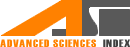
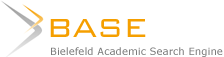

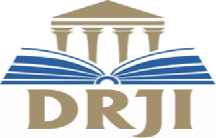

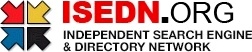

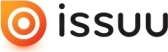

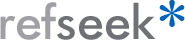
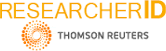
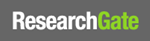
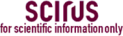
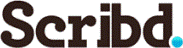

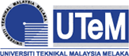
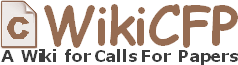
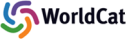
Federate Machine Learning: A Secure Paradigm for Collaborative AI in Privacy-Sensitive Domains
Author(s) | Kartheek Kalluri |
---|---|
Country | United States |
Abstract | Federated machine learning (FML) is a disruption-oriented paradigm of feature development in artificial intelligence (AI). This has posed the traditional query of data privacy, whether one really complies with both and, hence, working in the sensitive areas of health care, finance, and the IoT (Internet of Things). FML enables decentralized training of AI models instead of the traditional centralized AI framework: it allows the use of several local devices or even servers whereby raw data remains in a local site while collaborative learning can be harnessed. It ensures that data is always secured through state-of-the-art privacy-preserving techniques such as differential privacy, secure multiparty computation, and homomorphic encryption during training and aggregation. It, therefore, implies the application of distributed architectures, federated optimization algorithms like Federated Averaging (Fed Avg), along iterations for continuance improvement. Activity-specific refinements have proved the operational applicability of increasing diagnostic capability in health care, enhancing fraud detection in finance, and adopting privacy-sensitive functionality for smart devices in the IoT. How much FML cares for ethical AI development is demonstrated not only by the adherence to legally binding obligations such as the GDPR but also by HIPAA. Empirical results show that considerable improvement has been achieved in the areas of privacy protection and model performance and scalability without affecting data security or stakeholder trust. Research opportunities have been identified for communication overhead, bias mitigation, and adaptive scalability. In essence, FML erects a complete and strong, privacy-first framework for ethical, scalable, and collaborative development of AI in parallel to evolving innovation with society and in line with regulatory requirements on use. |
Keywords | Federated Machine Learning (FML), Data Privacy and Security, Privacy-Preserving Techniques, Distributed AI Frameworks, Federated Averaging (Fed Avg), Ethical AI Compliance, Scalability and Complex Mitigation |
Published In | Volume 13, Issue 4, October-December 2022 |
Published On | 2022-10-05 |
Cite This | Federate Machine Learning: A Secure Paradigm for Collaborative AI in Privacy-Sensitive Domains - Kartheek Kalluri - IJSAT Volume 13, Issue 4, October-December 2022. DOI 10.5281/zenodo.14551746 |
DOI | https://doi.org/10.5281/zenodo.14551746 |
Short DOI | https://doi.org/g8wtfg |
Share this

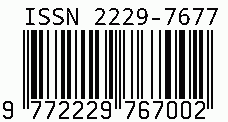
CrossRef DOI is assigned to each research paper published in our journal.
IJSAT DOI prefix is
10.71097/IJSAT
Downloads
All research papers published on this website are licensed under Creative Commons Attribution-ShareAlike 4.0 International License, and all rights belong to their respective authors/researchers.
