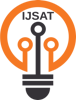
International Journal on Science and Technology
E-ISSN: 2229-7677
•
Impact Factor: 9.88
A Widely Indexed Open Access Peer Reviewed Multidisciplinary Bi-monthly Scholarly International Journal
Plagiarism is checked by the leading plagiarism checker
Call for Paper
Volume 16 Issue 2
2025
Indexing Partners

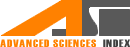
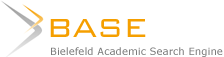

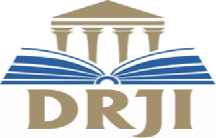

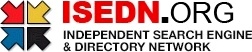

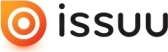

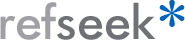
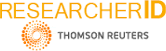
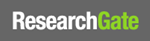
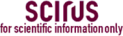
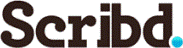

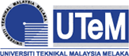
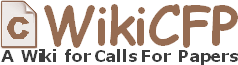
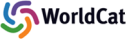
Machine Learning Approaches for Improving Supply Chain Efficiency and Demand Prediction
Author(s) | Perumallapalli Ravikumar |
---|---|
Country | United States |
Abstract | Machine learning (ML) has proven crucial in recent years for improving demand forecast models and increasing supply chain efficiency. The inherent problems of shifting demands, supply variability, and information asymmetry in supply chains may be resolved with the use of machine learning techniques. Traditional methods like ARIMA modelling and strategic contracting have provided fundamental insights as the supply chain ecosystem becomes more complicated, but sophisticated machine learning applications have started to offer more sophisticated prediction capabilities. This includes assessing effects such as the bullwhip phenomenon, which emphasizes the amplification of demand unpredictability across supply chain levels, and optimizing the placement of safety stocks.By integrating real-time data and allowing for dynamic adjustment to demand signals, machine learning models improve current tactics and lessen the negative effects of supply chain interruptions on operational performance. For sectors like fashion retail, where precise demand forecasting and inventory testing greatly enhance decision-making, these developments are especially beneficial. ML techniques assist optimize inventory levels and reduce lead times by incorporating demand data into replenishment models, improving overall responsiveness.Furthermore, ML's collaborative potential is revolutionary, particularly in situations where information sharing lowers risk and enhances supply chain partner alignment. For instance, it has been demonstrated that ML algorithms promote resilience against supply chain disruptions through insights into risk management, information exchange, and adaptive measures. Predictive modelling for system utilization and performance optimization and adaptive learning in response to real-time demand input are other benefits of the transition to AI-driven supply chain intelligence.As these approaches continue to advance, ML-based solutions are becoming increasingly important in enabling flexible and effective supply chains that are better able to manage present demands as well as upcoming difficulties, providing notable competitive benefits in a range of industries. |
Keywords | Supply Chain Efficiency, Demand Prediction, Inventory Optimization, ARIMA Model, Machine Learning in Supply Chains, Neural Networks (ANNs, RNNs), Reinforcement Learning, Bullwhip Effect Mitigation, Time-Series Forecasting |
Field | Computer Applications |
Published In | Volume 1, Issue 2, April-June 2010 |
Published On | 2010-04-05 |
Cite This | Machine Learning Approaches for Improving Supply Chain Efficiency and Demand Prediction - Perumallapalli Ravikumar - IJSAT Volume 1, Issue 2, April-June 2010. |
Share this

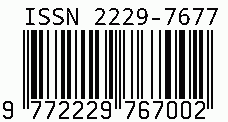
CrossRef DOI is assigned to each research paper published in our journal.
IJSAT DOI prefix is
10.71097/IJSAT
Downloads
All research papers published on this website are licensed under Creative Commons Attribution-ShareAlike 4.0 International License, and all rights belong to their respective authors/researchers.
