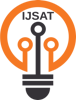
International Journal on Science and Technology
E-ISSN: 2229-7677
•
Impact Factor: 9.88
A Widely Indexed Open Access Peer Reviewed Multidisciplinary Bi-monthly Scholarly International Journal
Home
Research Paper
Submit Research Paper
Publication Guidelines
Publication Charges
Upload Documents
Track Status / Pay Fees / Download Publication Certi.
Editors & Reviewers
View All
Join as a Reviewer
Reviewer Referral Program
Get Membership Certificate
Current Issue
Publication Archive
Conference
Contact Us
Plagiarism is checked by the leading plagiarism checker
Call for Paper
Volume 16 Issue 1
2025
Indexing Partners

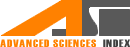
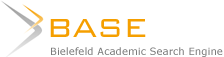

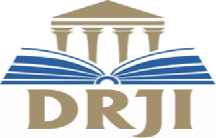

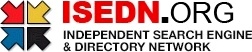

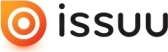

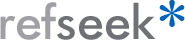
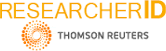
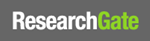
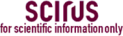
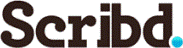

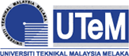
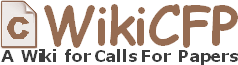
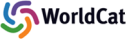
Predictive Modeling of Cryptocurrency Price Movements Using Autoregressive and Neural Network Models
Author(s) | Sandeep Yadav |
---|---|
Country | United States |
Abstract | Cryptocurrency markets are highly volatile and driven by complex, non-linear dynamics, posing significant challenges for price prediction. This research explores the predictive modeling of cryptocurrency price movements by integrating traditional statistical techniques, such as Autoregressive (AR) models, with advanced Neural Network (NN) architectures. The study evaluates the performance of these models in forecasting short-term price trends for major cryptocurrencies like Bitcoin, Ethereum, and Binance Coin. The dataset consists of historical price data and technical indicators, preprocessed to address missing values, outliers, and non-stationarity. Autoregressive models provide interpretable baseline predictions by capturing temporal dependencies in price series, while Neural Networks leverage their capability to learn complex patterns and relationships within the data. Experimental results demonstrate that Neural Network models, particularly Long Short-Term Memory (LSTM) networks, outperform AR models in terms of accuracy, root mean squared error (RMSE), and directional accuracy. However, AR models exhibit competitive performance in periods of low market volatility due to their simplicity and robustness. This research highlights the strengths and limitations of both approaches, providing insights into their applicability for cryptocurrency price prediction. The findings underscore the potential of hybrid models, combining the interpretability of AR models with the predictive power of NNs, to enhance decision-making in cryptocurrency trading and risk management. Future work could explore the integration of alternative data sources, such as social media sentiment and blockchain activity, to further improve prediction accuracy. |
Keywords | Cryptocurrency Price Prediction, Autoregressive Models, Neural Networks, Long Short-Term Memory (LSTM), Time Series Analysis, Price Forecasting, Machine Learning in Finance, Cryptocurrency Volatility, Predictive Modeling, Hybrid Prediction Models |
Published In | Volume 14, Issue 1, January-March 2023 |
Published On | 2023-01-09 |
Cite This | Predictive Modeling of Cryptocurrency Price Movements Using Autoregressive and Neural Network Models - Sandeep Yadav - IJSAT Volume 14, Issue 1, January-March 2023. DOI 10.5281/zenodo.14288541 |
DOI | https://doi.org/10.5281/zenodo.14288541 |
Short DOI | https://doi.org/g8txvq |
Share this

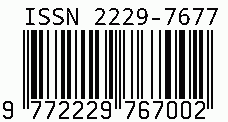
CrossRef DOI is assigned to each research paper published in our journal.
IJSAT DOI prefix is
10.71097/IJSAT
Downloads
All research papers published on this website are licensed under Creative Commons Attribution-ShareAlike 4.0 International License, and all rights belong to their respective authors/researchers.
